Enhancing Demand Planning with Predictive Analytics
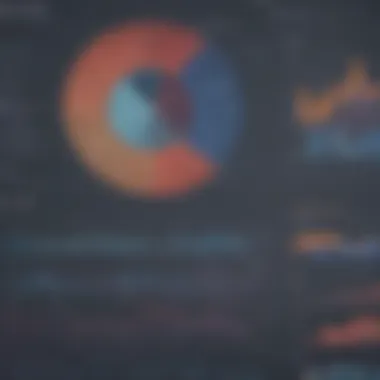
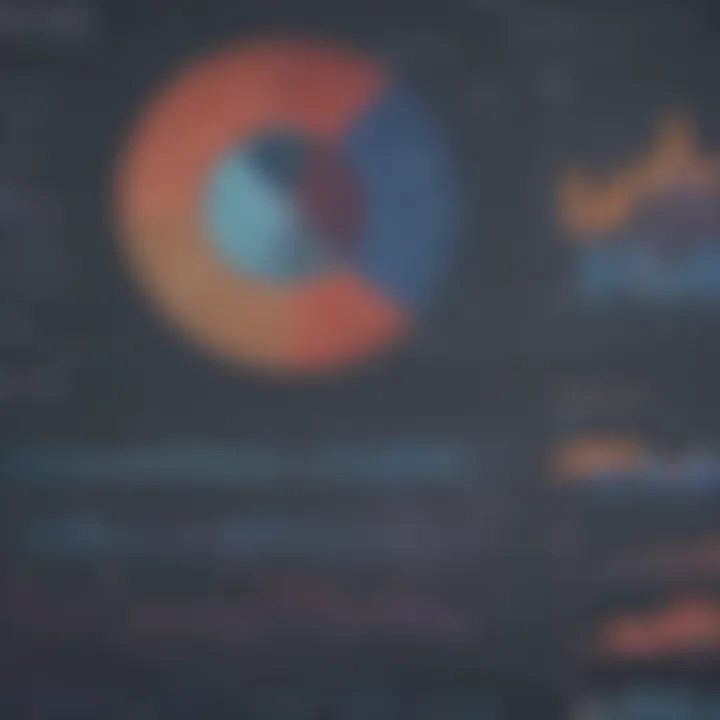
Intro
Predictive analytics is like having a crystal ball in the world of demand planning. In a landscape that's always shifting, organizations have turned to historical data and sophisticated algorithms to make sense of the chaos around customer behaviors and inventory needs. The art of effectively anticipating demand isn't as straightforward as flipping a switch; it requires finesse and understanding of the nuances of data. This discussion digs beneath the surface to explore how predictive analytics enhances demand planning, revealing actionable insights along the way.
One could argue that understanding customer behavior is similar to uncovering a treasure map. Each data point is a clue that leads companies to more informed decisions—whether that's optimizing inventory levels or fine-tuning production schedules. In a market where the only constant is change, the ability to predict demand can serve as a lifeline.
As we delve deeper, we’ll dissect the methodologies employed in predictive analytics, the real-world implications for demand planning, and the challenges that often arise. The goal is not just to skim the surface; rather, we aim to provide a thorough understanding of how predictive analytics—when done right—transforms the processes that govern how products are produced and delivered.
The key players in this space range from retail giants like Walmart to tech companies like Amazon, all latching onto predictive analytics as a means to stay ahead of the game.
"In the world of demand planning, being reactive is no longer enough—predictive analytics takes you a step beyond, allowing you not just to react but to preemptively navigate market shifts."
Through this article, we will piece together a narrative that offers students, investors, advisors, managers, and analysts a comprehensive understanding of why predictive analytics matters in the modern business landscape.
Understanding Predictive Analytics
Understanding predictive analytics is not just an intellectual exercise; it’s about grasping how data-driven insights can significantly impact decision-making in demand planning. This section sheds light on the value that comes from predictive analytics, which can be likened to having a compass in the oft-turbulent seas of market demand. With the right tools and techniques, organizations can anticipate customer behaviors and trends, allowing for more proactive rather than reactive strategies.
Predictive analytics harnesses the power of historical data and advanced computational techniques to forecast future outcomes. By doing so, it enables businesses to optimize their inventory management, refine production scheduling, and ultimately, improve overall operational efficiency. When organizations understand how to interpret and act on predictions, they stand to gain a substantial competitive edge.
Definition of Predictive Analytics
At its core, predictive analytics refers to the use of statistical algorithms and machine learning techniques to identify the likelihood of future outcomes based on historical data. The goal is clear: utilizing past data to foresee what might come next, thus empowering companies to strategize efficiently.
For example, a retail store may analyze previous sales records to predict customer preferences during the holiday season. This foresight informs decisions about how much inventory to keep on hand. Notably, predictive analytics can span various domains; it’s not limited to retail. Manufacturing, healthcare, and finance industries leverage these techniques to forecast everything from market trends to equipment maintenance needs.
Historical Context and Evolution
The history of predictive analytics is as rich as the data it utilizes. It isn’t a recent phenomenon but has evolved substantially over the decades. In the early days, organizations relied predominantly on basic statistical methods and intuition for forecasting. As data collection methods advanced throughout the 20th century, so did the techniques for interpreting that data.
The rise of computing power in the late 20th and early 21st centuries has revolutionized predictive analytics. Algorithms that once took days to process can now deliver insights in real time. The advent of machine learning has introduced more sophisticated models capable of identifying patterns that are sometimes too complex for manual analysis. Nowadays, predictive analytics not only includes traditional statistical methods, but also deeper learning approaches that mimic human decision-making patterns. This evolution opens doors for businesses to harness vast amounts of data, turning it into a gold mine of actionable insights.
"The implementation of predictive analytics is akin to navigating with a map and compass in a fog; it illuminates the way forward, but requires careful interpretation."
Key Components of Demand Planning
Demand planning stands as a cornerstone in the strategic operations of businesses, particularly in sectors where stock levels and production schedules can make or break success. To delve deeper into this field, we will look into three crucial components: forecasting demand, inventory management, and production scheduling. Each element plays a vital role in shaping an organization’s response to market demands and ultimately, its bottom line.
Forecasting Demand
Forecasting demand is often considered the backbone of any effective demand planning strategy. This process involves predicting customer demand based on historical sales data and market trends. Organizations utilize various methods, from simple moving averages to complex statistical analyses, to forecast sales figures.
One of the prominent benefits of accurate forecasting is the reduction of overstock and stockouts—both of which can be costly to a business. A company that can predict demand accurately can allocate resources efficiently, ensuring that production levels align with consumer needs. Furthermore, employing predictive analytics allows businesses to factor in external variables like economic indicators, seasonality, and marketing campaigns.
Research indicates that companies that engage in robust forecasting achieve an average of 10-20% improvement in forecasting accuracy. Such enhanced precision is pivotal in building trust with customers, as it leads to better fulfillment rates and ultimately, enhanced customer satisfaction. Here are some key points in demand forecasting:
- Utilizes historical data to predict future sales.
- Improves inventory turnover rates.
- Aligns marketing efforts with anticipated demand.
"Accurate demand forecasts can serve as a compass, guiding companies through the uncertain waters of market demands and consumer preferences."
Inventory Management
Inventory management swiftly follows demand forecasting as another crucial component of demand planning. This aspect involves overseeing the flow of goods from manufacturers to warehouses and ultimately to the point of sale. It’s not merely about knowing what’s on the shelves—efforts also include determining the right amount to stock, where to store it, and when to reorder.
A business that masters its inventory management practices can experience significant cost savings and improved cash flow. For instance, a just-in-time model, grounded in predictive analytics, enables firms to maintain minimal stock levels without risking running out of product.
Important considerations in effective inventory management include:
- Regular audits to assess stock levels and needs.
- Point-of-sale data that informs order quantities.
- Utilization of software tools designed for inventory tracking.
An added advantage of effective inventory management is that it provides a clearer insight into working capital, allowing for strategic investment in other areas of the business. Companies that invest in these tools often see a notable decrease in holding costs and waste.
Production Scheduling
The final component is production scheduling, which is all about timing and coordination. Scheduling production refers to managing the sequence and timing of operations to meet the predicted demand. Predictive analytics plays a significant role here, ensuring production lines run efficiently and responsively to fluctuations in demand.
A well-designed production schedule considers machinery availability, workforce management, and raw material supply. Organizations that effectively harness these elements can reduce turnaround times and enhance overall efficiency. This is particularly important in industries where delays can lead to increased costs and unresolved customer orders.
Benefits of robust production scheduling include:
- Minimized machine downtime through effective planning.
- Reduction in labor costs associated with overstaffing or last-minute adjustments.
- Enhanced collaboration with suppliers to ensure materials are available when needed.
Methodologies in Predictive Analytics
The methodologies in predictive analytics form the backbone of how organizations approach demand planning. Choosing the right method is crucial, as it not only influences the accuracy of forecasts but also impacts operational efficiency. These methodologies can harness a myriad of data sources, allowing companies to shift their strategies based on trends and patterns. With the ever-changing landscape of market dynamics, being well-versed in various methodologies ensures that businesses stay ahead of the curve.
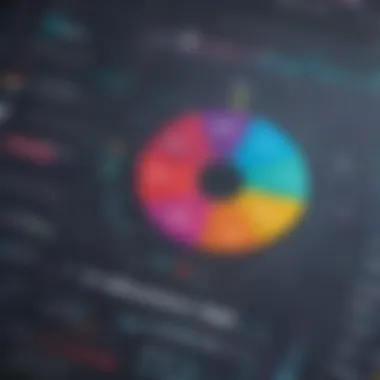
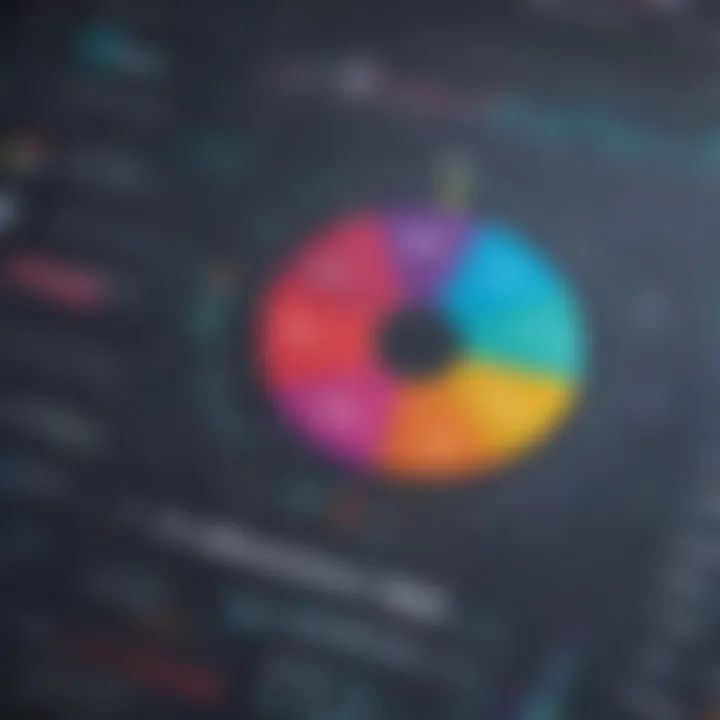
Statistical Techniques
Statistical techniques are fundamental in predictive analytics. They allow for the interpretation of historical data while helping to discern the relationships between different variables. Methods like regression analysis, time series analysis, and ARIMA (AutoRegressive Integrated Moving Average) offer a structured approach to identifying trends.
- Regression Analysis: This technique helps in identifying how the dependent variable changes when one or more independent variables vary. For example, a retailer may use regression to predict sales based on advertising spend, pricing changes, and seasonal demands.
- Time Series Analysis: This technique focuses specifically on data points collected or recorded at specific time intervals. Businesses often apply this to analyze sales performance over seasons or uncover patterns in consumer behavior across time.
- ARIMA Models: These are used when analyzing time series data that show trends over time. They are particularly useful when the data has seasonality and trends, making it essential for sectors like retail that experience fluctuations based on seasons.
Statistical techniques allow organizations to derive insights from past performance data, thereby informing future strategies.
Machine Learning Applications
Machine learning plays an increasingly pivotal role in predictive analytics, especially when it comes to making real-time predictions. By leveraging algorithms that learn from data patterns, organizations can refine their models continuously as new data becomes available.
- Supervised Learning: This involves training a machine learning model on a labeled dataset. When predicting future sales based on historical data, algorithms like decision trees or support vector machines can produce incredibly accurate forecasts.
- Unsupervised Learning: In this case, models can identify hidden patterns in data without pre-defined labels. For example, customer segmentation can be improved through clustering algorithms, helping businesses tailor their products and marketing efforts based on consumer behavior.
- Predictive Modeling with Neural Networks: This complex approach mimics human brain function to predict outcomes. It’s particularly useful in industries with large datasets, like e-commerce, where neural networks can analyze patterns in purchasing behavior.
Machine learning methodologies not only enhance the precision of forecasts but also adapt the models to new input data, ensuring ongoing improvement in the prediction process.
Data Mining Approaches
Data mining acts as the bridge between raw data and actionable insights. It employs various techniques to dig through extensive datasets to discover patterns and trends that can boost demand planning efforts.
- Association Rule Learning: This technique identifies interesting relationships between different variables in large databases. For instance, it could reveal that customers who buy laptops are also prone to purchase printers. Retailers can use this data to create bundled offers.
- Sequential Pattern Mining: Useful in understanding customer shopping behavior over time, this technique analyses sequences of purchases. For example, recognizing the patterns of consumers who typically buy winter clothing before holiday seasons can help forecast demand accurately.
- Anomaly Detection: This identifies data points that deviate from a standard pattern. Detecting unusual spikes in sales can offer insights into external factors affecting demand, such as societal trends or economic changes.
Data mining approaches empower organizations to sift through informational noise, isolating vital insights that directly influence demand planning decisions.
"In the realm of predictive analytics, the methodologies implemented can make all the difference between success and failure in demand planning strategies."
By employing these methodologies—statistical techniques, machine learning applications, and data mining approaches—organizations can effectively navigate the complexities of demand planning, ultimately enhancing their adaptability and operational smoothness.
Data Sources for Predictive Analytics
In the realm of predictive analytics, the data sources function as the very bedrock upon which sound decision-making is built. Without rich, meaningful data, the algorithms employed can only go so far in providing accurate insights. Organizations stand to benefit significantly by tapping into a variety of internal and external data sources that can enhance their demand planning efforts. Understanding varying data sources is crucial not only for optimizing the analytic process but also for tailoring business strategies that can respond to market fluctuations effectively.
The right data can illuminate the paths that lead to better forecasting accuracy, improved resource management, and ultimately help a business stay one step ahead of the competition. Companies often find themselves swimming in a sea of data, and knowing where to fish for useful nuggets becomes paramount.
"Data is the new oil; it’s valuable, but if unrefined it can’t really be used."
Internal Data Collection
Internal data collection refers to the gathering of information from within the organization's own systems. This might include transaction records, inventory levels, customer feedback, and sales data. Think of it like sifting through your personal history for clues that will help predict future behavior. Here’s why internal data is indispensable:
- Accuracy and Relevance: Internal data is often cleaner and more accurate compared to external sources. By utilizing this data, businesses can maintain a tighter grasp on what’s happening within their operations.
- Timeliness: Accessing real-time data enables organizations to spot trends as they arise, rather than being caught off guard by historical patterns that may no longer apply.
- Comprehensiveness: Internal metrics provide a holistic view of operations. For instance, combining sales data with inventory levels can help ensure optimal stock levels are maintained to meet demand without overcommitting resources.
However, firms must remain vigilant about the potential pitfalls of relying solely on internal data. It is important to continually assess the impact of external variables that may not be captured within the organization, such as market trends or competitive behaviors.
External Data Integration
External data integration encompasses data sourced from outside the organization. This may include market reports, social media sentiment, economic indicators, and even competitor analysis. This type of data enriches the predictive model considerably. The incorporation of external data can lead to several advantages:
- Broader Insight: Relying only on internal data can lead to a narrow view of the market. External data provides context, helping teams understand larger trends affecting demand.
- Enhanced Forecasting: Merging internal data with external indicators enables more robust predictions. For example, a retail company may supplement sales data with data on economic trends to forecast seasonal demand more accurately.
- Innovation and Adaptability: Staying informed about changes in the external environment—like shifts in consumer preferences or emerging market segments—can facilitate flexibility in demand plans.
Integrating external data can, however, present challenges. Issues with data quality, discrepancies in measuring units, and the volume of data can overwhelm analytical processes. Therefore, companies must prioritize not just the collection, but also the integration of external data into their existing frameworks to ensure it adds real value.
Implementation in Demand Planning
Implementing predictive analytics in demand planning is like laying the foundation of a sturdy house. This foundation supports everything above and ensures that the structure stands tall against uncertainties in the market. By embracing predictive analytics, companies can leverage historical data to bolsters decision-making processes.
For one, organizations can develop a predictive model that reflects their unique operational realities. This model helps in forecasting demand with greater precision, thus informing inventory management, production scheduling, and other critical aspects involved in demand planning.
The benefits here are numerous:
- Enhanced accuracy in forecasting leads to better stock levels, reducing excess inventory and related costs.
- Improved resource allocation allows companies to streamline operations, ensuring resources are diverted where they are needed most.
- Ultimately, these efficiencies contribute to substantial cost savings and improved customer satisfaction.
However, implementing predictive analytics isn't a walk in the park. Organizations must address several considerations to ensure success. First, the quality of data plays a pivotal role. Without accurate data, it is impossible to develop a reliable predictive model. Additionally, there must be a cultural shift within organizations to embrace data-driven decision-making.
Developing a Predictive Model
When developing a predictive model, the focus is to identify variables that influence demand accurately. This requires a thorough understanding of historical sales data, customer behavior, and market conditions. Companies often start by gathering a breadth of data, which may come from internal databases that track sales performance and customer interactions, as well as external sources, such as market trends and competitor analyses.
A critical step is to use appropriate statistical techniques and algorithms to create a robust model. Some factors to consider include:
- The seasonality of products, which helps determine peak times for inventory needs.
- Promotional activities that could lead to spikes in demand.
- Trends in consumer behavior, which often shift due to economic conditions or changes in consumer preferences.
"Building a predictive model is akin to piecing together a puzzle. Each data point provides a clue that helps reveal the broader picture of consumer demand."
Once the model is built, it needs to be calibrated continually to stay relevant to changing market dynamics.
Testing and Validation
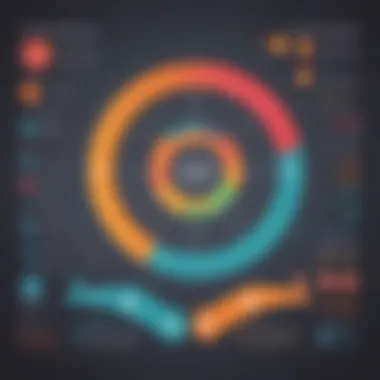
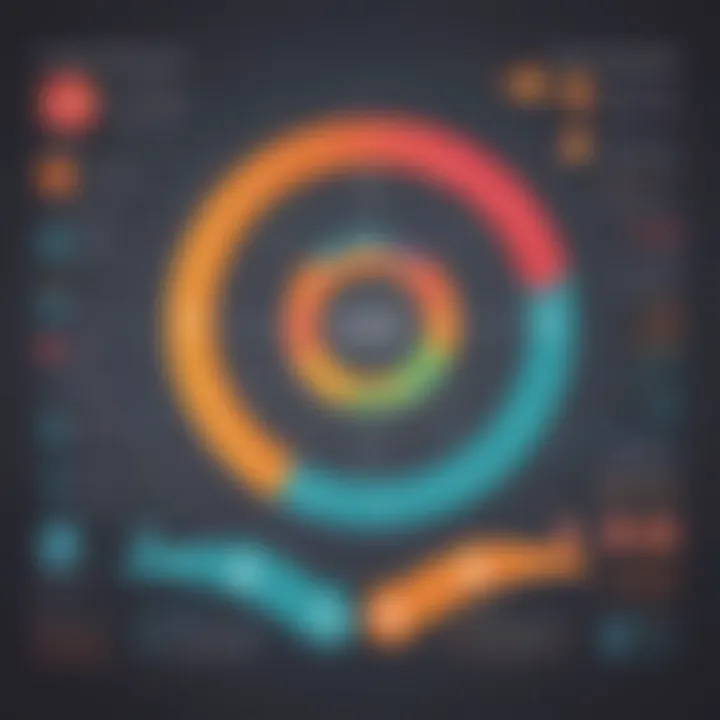
Testing and validation are equally important steps in the predictive analytics journey. After developing a model, organizations must rigorously evaluate its effectiveness. This process often involves comparing the model's forecasts against actual sales data over a defined period.
Several methods are available for this:
- Backtesting involves applying the model to historical data to see how well it could have performed.
- Statistical tests assess the model’s accuracy. Measures such as MAE (Mean Absolute Error) or RMSE (Root Mean Squared Error) can illustrate how close forecasts come to actual values.
Once validated, it’s critical to apply insights drawn from testing. This might mean tweaking the model parameters, employing different algorithms, or adjusting the input data sets. The aim here is to ensure that the model is as reliable and insightful as possible, generating value for demand planning efforts.
Integration with ERP Systems
Integrating predictive analytics into existing Enterprise Resource Planning (ERP) systems is the next step that carries immense significance. This integration ensures that the power of predictive analytics is harnessed throughout the organization.
In practical terms, this means that predictions generated from the analytical models can directly inform operations across various departments such as marketing, supply chain, and finance. There are several factors to consider during this integration:
- Data compatibility: Ensuring that data formats and structures are uniform across systems is crucial for smooth functioning.
- User access: Providing relevant stakeholders with access to insights without over-complicating their workflows encourages the uptake of predictive analytics throughout the organization.
- Continuous learning: Establishing a feedback loop within the ERP system allows for real-time adjustments based on new data coming in.
In essence, through proper integration, predictive analytics can become a vital component of the decision-making apparatus, unlocking the full potential of demand planning while promoting a more agile operational strategy.
Benefits of Predictive Analytics in Demand Planning
Predictive analytics is fundamentally reshaping how companies approach demand planning. This isn't just about keeping up with customer demands. It's about getting ahead of them, foreseeing trends, and making strategic decisions that can lead to a competitive edge. The benefits of predictive analytics are numerous, covering enhanced forecasting accuracy, improved resource management, and effective cost-saving strategies.
When businesses harness the power of predictive analytics, they can truly understand the patterns in consumer behavior and market trends. It's like having a crystal ball – a glimpse into what the future holds. Organizations can ensure that they have the right products in stock at the right time, which ultimately improves customer satisfaction and drives sales.
Improved Accuracy in Forecasting
Forecasting demand accurately is a nuanced process. By utilizing historical data with advanced analytical tools, businesses can generate forecasts that are more precise and reliable. Instead of relying solely on intuition or past sales figures, companies employ statistical models and machine learning to analyze trends, seasonality, and external factors.
For example, an online clothing retailer may notice a seasonal increase in coats every winter. Using predictive analytics, they can factor in weather forecasts and local events to predict the exact demand. This results in fewer stockouts and overstock situations, allowing retailers to serve customers better while maximizing profitability.
"The closest guess can still miss the mark; predictive analytics sharpens the dart."
Enhanced Resource Allocation
Resource allocation is another critical area where predictive analytics shines. With accurate forecasts, businesses can allocate their resources – be it workforce, technology, or materials – more effectively. This aids not only in fulfilling customer orders promptly but also ensures that resources are not wasted on unnecessary inventory or labor costs.
Take a manufacturing plant that experiences fluctuating demand for its products. By analyzing data trends, they can adjust staffing levels according to forecasted demand. This not only prevents overstaffing during slow periods but also mitigates the risks associated with sudden demand spikes. When resources are aligned with actual demand, the entire operation runs smoother.
Cost Reduction Strategies
Finally, cost reduction strategies closely tie into both accurate forecasting and resource allocation. Companies can significantly cut their operational costs by reducing excess inventory, which often comes with holding costs that can eat into profit margins.
Identifying trends in consumer behavior allows companies to make smarter purchasing decisions. For instance, if a salad dressing manufacturer knows that their products are trending down in popularity, they can cut back on production ahead of time instead of facing the grim reality of surplus stock at the end of the season.
Furthermore, predictive analytics can help in negotiating better terms with suppliers. By providing data-backed forecasts, companies can plan for bulk orders when necessary, ensuring they leverage pricing dynamics.
In summary, the benefits of predictive analytics in demand planning are significant. With improved accuracy, enhanced resource allocation, and effective cost strategies, businesses can navigate the complexities of the market with confidence, positioning themselves favorably for sustained growth.
Challenges and Limitations
The integration of predictive analytics in demand planning is often met with various challenges and limitations. It’s crucial to address these hurdles since they can impede the effectiveness of the analytics processes. Without acknowledging these issues, organizations might misjudge the capabilities of predictive models, leading to poor decision-making. Understanding these challenges helps managers and analysts devise strategies to minimize obstacles while maximizing the analytical insights they glean.
Data Quality Issues
When it comes to predictive analytics, one of the biggest stumbling blocks is undoubtedly data quality. Managers need to ensure that the data being collected is reliable, accurate, and relevant. After all, garbage in, garbage out holds true in this context. If data records are riddled with inconsistencies or inaccuracies, the predictions derived from such data will likely be unreliable.
Take for instance a retail company that relies on customer purchase history to forecast demand. If the data lacks proper categorization or has missing records, the predictions made will be less accurate. Inaccurate data can lead to overstocking or understocking, creating a domino effect of inefficiencies. To combat this, organizations should invest in effective data cleansing and validation processes. Regular audits of the data ensure that only high-quality information is utilized for predictive modeling.
Resistance to Change in Organizations
Change is an integral part of any industry, yet it often meets resistance, especially when it comes to adopting new technologies like predictive analytics. Employees may feel threatened by new tools, fearing their roles may become redundant. Some may resist changing their established ways of working, even if those methods are less efficient.
For instance, a manufacturing unit that has relied on traditional demand planning methods may hesitate to adopt data-driven predictive analytics due to skepticism surrounding the technology. This resistance can stall the integration process and diminish overall organizational efficiency. To overcome these barriers, management must create a culture that embraces change. Providing training and demonstrating the tangible benefits of predictive analytics can help in assuaging concerns. Encouraging employees to share their perspectives might also foster a sense of ownership and reduce reluctance.
Complexity of Models
Many predictive analytics models can be quite complex, requiring sophisticated algorithms and deep knowledge of statistics and data science. This complexity can be a double-edged sword. On one hand, advanced models can yield precise and nuanced predictions. On the other hand, they can also alienate those without specialized skills.
Consider a scenario where a firm implements a complicated machine learning algorithm for demand forecasting. If the team handling this model lacks the necessary expertise, they might struggle to interpret the results or, worse, misapply the insights derived. This is where the balance between complexity and usability becomes essential. Organizations should aim for models that align with their team’s capabilities. Offering training sessions or collaborating with data science experts can lead to better understanding and ultimately more effective use of predictive analytics.
"One of the first steps in effective predictive analytics is not just gathering data but also ensuring that the data is accurate and well-structured for analyses."
In summary, acknowledging the challenges and limitations of predictive analytics in demand planning is the first step towards effective implementation. Fostering a culture that values data integrity, actively addressing resistance to change, and carefully managing model complexity are pivotal to overcoming these barriers. Organizations that navigate these challenges effectively will find themselves better positioned to harness the full power of predictive analytics.
Real-world Applications
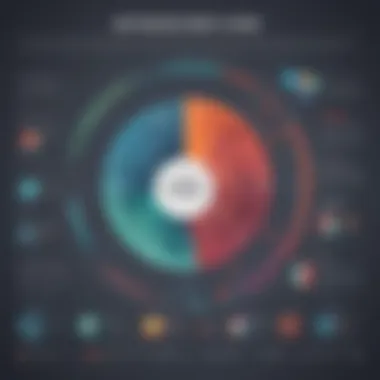
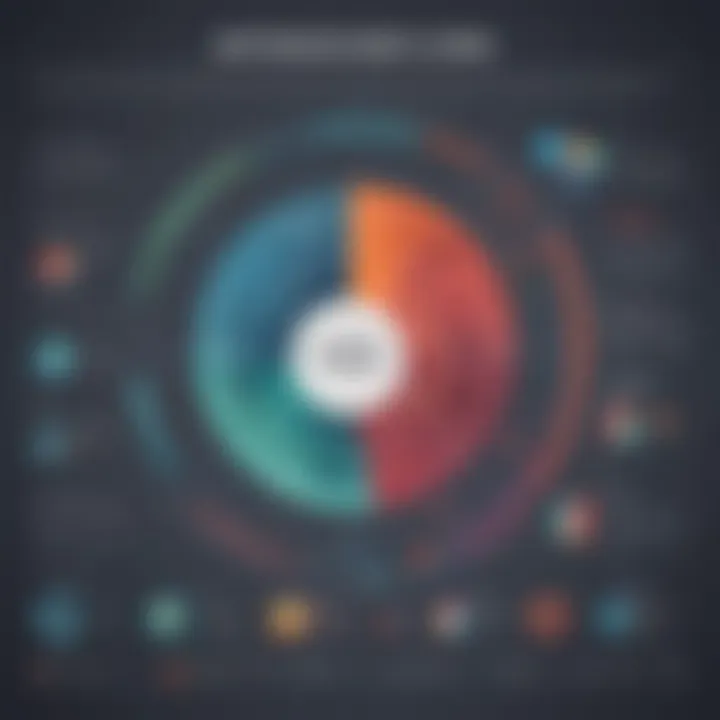
Understanding how predictive analytics works in various sectors is vital for industry professionals. It provides insights into consumer behavior, enhances decision-making, and enables organizations to stay competitive. In essence, the real-world applications of predictive analytics extend far beyond theoretical frameworks and play a crucial role in daily business operations.
Retail Industries
In the retail sector, predictive analytics has transformed how companies approach inventory management and customer engagement. By analyzing historical sales data, retailers can forecast which products will be in demand during specific seasons or events. For example, during back-to-school season, a clothing retailer may notice an uptick in sales of certain styles based on previous years’ data. This allows for timely adjustments in stock levels to meet forecasted demand.
Moreover, sophisticated algorithms can also help retailers in targeted marketing. By using customer purchase history and preferences, these algorithms can create personalized offers, enhancing customer experience and ultimately driving sales. It’s akin to knowing your customers better than they know themselves. However, to be effective, companies must ensure that the data being used is accurate and relevant.
Manufacturing Sectors
In manufacturing, predictive analytics shines a light on operational efficiencies. By employing these techniques, manufacturers can predict equipment failures before they happen. This predictive maintenance can save time and significantly reduce costs associated with unplanned downtime. For instance, a manufacturer of automotive parts can analyze patterns in machinery operation to foresee potential breakdowns, allowing for proactive repairs.
Additionally, demand forecasting in manufacturing leads to better production scheduling. By aligning production with expected demand, companies can avoid both overproduction and stockouts. They benefit from an agile approach, enabling a quick response to market changes. The combination of accurate data analysis and timely production decisions leads not only to a more streamlined process but also higher profit margins.
E-commerce Platforms
E-commerce platforms are perhaps the most dynamically impacted by predictive analytics. They rely heavily on real-time data to drive decisions and improve customer interactions. By analyzing users’ online behaviors—like click patterns and item views—e-commerce businesses can craft a personalized shopping experience. When a customer frequently browses a particular type of laptop, the website might feature related products prominently, increasing the chances of a sale.
Additionally, having predictive insights allows e-commerce businesses to optimize their supply chain. Companies can analyze trends in product sales to ensure that they have adequate stock without unnecessary excess. This approach helps reduce storage costs and minimizes waste, as products can quickly go out of style in a fast-paced market.
"Predictive analytics enables e-commerce businesses not only to meet customer demands but to anticipate them, often leading to high levels of customer satisfaction."
In summary, regardless of the industry, predictive analytics offers substantial benefits. By implementing these techniques, businesses foster better decision-making, boost operational efficiency, and cater more effectively to consumer demands, all of which contribute to long-term success.
Future Trends in Predictive Analytics
As the landscape of demand planning continues to evolve, understanding the future trends in predictive analytics becomes increasingly vital. Organizations are not only aiming to stay afloat amidst rapid changes but are also striving to harness the full potential of predictive analytics to elevate their operations. Below, we dissect several emerging trends that are shaping the future of this field, offering key insights for students, investors, advisors, managers, and analysts alike.
Artificial Intelligence Integration
The integration of artificial intelligence (AI) into predictive analytics marks a leap forward in how data is processed and insights are generated. Unlike traditional data analysis methods, AI algorithms can adapt and learn from vast datasets, providing more nuanced predictions and eliminating guesswork. For instance, companies like Amazon depend on AI to predict purchasing behavior and stock levels, allowing them to adjust inventories with precision.
- Benefits of AI Integration:
- Improved accuracy in demand forecasting.
- Enhanced speed in data processing.
- The ability to analyze complex relationships in data that traditional methods might miss.
As organizations adopt AI, they’ll likely face the challenge of ensuring their workforce is equipped with the necessary skills to leverage these tools. Additionally, ethical considerations around data privacy and algorithm fairness will play an important role in guiding AI implementations in predictive analytics.
Growing Importance of Real-time Data
In today’s fast-paced business environment, the need for real-time data has never been higher. Predictive analytics thrives on timely information, which means businesses must prioritize integrating systems that offer up-to-the-minute insights. For example, companies in the grocery sector use real-time analytics to track consumer behaviors as they happen, adjusting stock levels accordingly to minimize waste and maximize sales.
- Key Aspects of Real-time Data:
- Enables immediate adjustments to demand plans, improving responsiveness.
- Supports a more dynamic inventory management process.
- Enhances customer experience by aligning product availability with actual consumer demand.
As more companies invest in IoT devices and advanced data capturing tools, the value of real-time insights will continue to soar. However, organizations must also ensure that they have robust data governance frameworks in place to mitigate risks associated with data quality and security.
Shift Towards Cloud Computing
Cloud computing is another pivotal factor driving change in predictive analytics. By utilizing cloud storage and processing power, businesses can access large volumes of data and sophisticated analytics capabilities without the hefty upfront costs of maintaining on-premise infrastructure.
- Advantages of Cloud Computing:
- Scalability: Easily adjust resources based on evolving needs.
- Cost-Effectiveness: Reduce the expenses tied to hardware and software maintenance.
- Collaboration: Enable teams to work together across geographies more seamlessly.
With major platforms like Google Cloud, Microsoft Azure, and AWS leading the space, companies have more options than ever to implement effective predictive analytics solutions. Transitioning to the cloud also brings challenges, including concerns over data security and compliance, which organizations must carefully navigate.
Prediction: As predictive analytics continues to evolve, the intersection of AI, real-time data, and cloud computing will redefine how organizations approach demand planning. The ability to quickly adapt to changes in consumer behavior while maximizing efficiency will become a competitive advantage, underscoring the importance of these emerging trends.
Understanding and adapting to these trends is crucial for decision-makers seeking to utilize predictive analytics effectively in their demand planning processes. There are vast opportunities ahead, ensuring that the industry remains at the cutting edge of technological advancements.
Ending
In concluding the discussion on predictive analytics in demand planning, it becomes clear that this discipline is not just a passing trend but a pivotal element in today's business landscape. The synthesis of data-driven decision-making offers numerous benefits, such as improved accuracy in forecasting, enhanced resource allocation, and ultimately, cost reduction. As organizations strive to align their operations with market demands, the implementation of predictive analytics becomes essential.
Summation of Key Points
In this discourse, several key points have emerged:
- Evolving Landscape: Predictive analytics has transformed dramatically over the years, integrating cutting-edge technologies such as machine learning and AI, which have diversified its applications.
- Strategic Resource Allocation: With refined forecasting techniques, businesses can allocate resources more efficiently, adapting their inventory and production schedules to actual demand rather than relying solely on historical data.
- Competitive Edge: As demand patterns continue to shift, organizations utilizing advanced predictive models stand a better chance at maintaining a competitive advantage by anticipating market needs rather than reacting to them.
Final Thoughts on Implementation
The journey of embedding predictive analytics within demand planning requires a strategic approach. First and foremost, organizations must prioritize data quality, ensuring that the inputs feeding into predictive models are accurate and up to date. Resistance to adopting new methods can be a hurdle, but effective change management and training can help elicit a company-wide shift in perspective.
It's also crucial to recognize the complexity of the models being developed. As businesses embrace this tool, ongoing testing and validation will be vital to clarify that the predictive outcomes align with real-world scenarios. With investments in the right technology and a focus on integration with existing systems, businesses can leverage predictive analytics not just as a functional tool, but as a backbone of their operational strategy.
Ultimately, the evolution of demand planning through predictive analytics holds significant promise, but reaping those benefits requires foresight, planning, and a willingness to adapt to the nuances of an ever-changing marketplace.
"In a world saturated with data, the ability to predict trends before they emerge becomes not just an advantage, but a necessity for survival."
The horizon looks bright for those who embrace this evolving landscape.